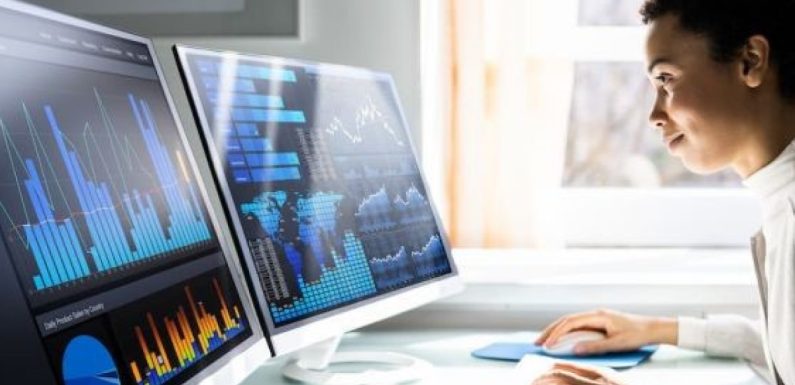
Predictive analytics empower financial decision-makers to envision more strategic approaches that mesh with business objectives. Backed by data governance standards related to those models, decision-makers can have more confidence in the quality and accessibility of the resulting scores.
Such tools can give financial professionals powerful insights that help them to make data-driven predictions, such as forecasting future cash flows, mitigating risks and optimising investment strategies, from predicting equipment downtime to optimising client retention strategies.
Predictive Models
A predictive model is a type of algorithm that takes data from the past and the outcomes of it to predict a future outcome. Examples of various predictive models include statistical algorithms and machine learning methods using regression models such as Polynomial, KNN, Random Forests and Linear Regression, as well as timing series methodologies to help to smooth out variations in the data such as Arima, Gaussian Process, KALMAN FILTER, Support Vector Regression and Smoothing Average models.
Through the website, a firm will then be able to predict with more precision than before, thus gaining a competitive edge. For example, the various factors that contribute to a delivery company being at its busiest could be calculated so that the management can hire more drivers, cut fuel costs and service delays far in advance of when they are scheduled.
Another popular application is healthcare (patient adherence to a treatment plan), then banking (high-risk or low-risk customer profile), and insurance (fraud). Predictive tools are super detectives for businesses; they recognise patterns and relationships that help make better use of resources, allowing companies to optimise efficiency and save money.
Time Series Models
Time series models learn from the input at a given time and the output at that time, then look at how the input has changed with respect to the output at the next time point, and so on. A time series model, which can be manually constructed or come from a machine learning algorithm, is part of the broader group of models that can be used to predict stock prices and other financial results.
Measuring past events this way allows time-series modelling to predict future demand for goods and services, for instance whether construction supplies companies need to prepare for a surge in buying activity, as predictive analytics tells them it will occur during hurricane season or immediately after hurricanes make landfall. They can thus adjust inventory levels and staffing levels to better serve their customers.
In the realm of health insurance, insurance companies and casinos use predictive analytics as one source of decision-making, and marketing companies also play a role. Predictive analytics can also forecast which patients will likely incur higher costs, as well as put at risk the lives of other patients. PSEG Long Island’s predictive analytics forecasts where future power outages are likely to occur hours ahead of time, then sends the appropriate number of crews and equipment prior to any loss of service. They can even prepare for the worst-case scenario, and be ready when a disaster strikes.
Regression Models
As such, regression models are essential to business analysts who extrapolate, and thereby attempt to predict, the future results from analysing data from the past, whether it is in supply chain management, customer acquisition, risk assessment and revenue projection, among others, all of which have a parallel in supply chain administration.
Most frequently utilized regression modeling in financial forecasting include:
Forecasting Demand: Many businesses rely on predictive analytics to understand what consumers are looking to buy, and the seasonality that is associated with consumer behaviour to project what is going to be bought in the coming weeks and months. Knowing that consumer demand will spike in spring or holiday seasons, such as the sales that uptick around the Christmas holiday, helps stores better predict how much of a particular good to stock and appropriately staff their store at the appropriate time. For example, construction stores know that sales will spike during and after hurricane season as homeowners repair their homes, so knowing this about consumer demand helps them to better manage inventory and staffing levels.
Better operations: Airline reservation systems and hotels use predictive analytics to forecast demand so they can set prices on tickets and rooms that will satisfy passenger demand with their available capacity. Predictive analytic allows businesses to optimise resource allocation to reduce risk and improve margins, for example by looking at the patterns in a customer’s actions such as a credit score or an insurance claim (one early us was Geisinger Health, the Pennsylvania hospital chain who used the technique to identify people most at risk of developing sepsis).
Moving Average Models
Predictive models use algorithms to identify relationships between data sets to estimate future numbers or events, delivering information that can be used to optimise an organisation’s operations, or guide decision-making, or maximise revenues or minimise risks.
For example, the use of predictive analytics spans a range of activities that include credit scores and insurance claims, debt collections and so on, all the way to regression analysis, finding the relationship between variables with the aim of predicting some value say a stock price or some measure of sales in order to be able to predict or forecast a particular desired value. There are several different ways to perform this regression analysis, some including use of machine learning techniques. Regression analysis is one of the most widely used forms of predictive analytics nowadays.
Moving average models provide another tool, where mistakes in the past are accounted for and used into the prediction of a quantity (be it, in this example, stock price, sale numbers or temperature) when issuing predictions for the future. These are fast, inexpensive models that readily account for missing values. They also readily scale to accommodate new data – the allergy sensor created by AbbieSense uses predictive analytics to warn of an allergic reaction before it occurs, and inject Epinephrine before humans can.